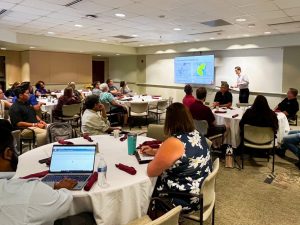
Salisbury, MD–On August 24, 2022, the City of Salisbury’s Information Services Department hosted members of the Maryland Governor’s office, regional law enforcement, and health and behavioral health providers at Salisbury University for an update and discussion on predictive modeling.
Predictive modeling, also called predictive policing, is the process of generating deep/machine learning algorithms to predict crime and behavioral patterns in order to address law enforcement needs and regional concerns.
This effort to integrate and develop predictive modeling began in 2018, when the City of Salisbury received a grant through the Governor’s Office of Crime Control & Prevention (GOCCP). Through this grant, the City’s Information Services Department began a project focused on addressing law enforcement needs at a municipal level more consistently than the current predictive policing models offered by national organizations.
“Initially, we were interested in reducing crime by using data to get ahead of crime through preventive efforts,” said Salisbury Police Department Chief Barbara Duncan. “Once we experienced success with commercially available predictive models, we began to look at whether predictive analytics might produce better outcomes if the analytics included non-traditional data sets which were more connected to the specific needs of our community. We realized that the best way forward would be to begin working on deep machine learning at the local level,” she added.
By July 2019, Grace Malcom, Data Scientist for the City of Salisbury, had completed a unique deep learning algorithm that exceeded the industry standard for accuracy (80%) in a law enforcement environment by consistently predicting the type, time, and place of crime at an 89% accuracy level. The project was then transferred back to the State for verification and further research, and in 2021, the University of Maryland took up the project.
“We believe that using predictive modeling at the municipal level is one of the most effective tools we have at our disposal to reduce crime in our City,” said Mayor Jake Day. “Predictive modeling uses a highly accurate and rigorous algorithm to aid our law enforcement officers in their incremental duties–of protecting and serving our residents, enforcing our laws, and overseeing safety in our communities. There is extensive evidence that predictive modeling is accurate and effective, and we are proud to be one of the key players at the forefront of these developments in our state,” he added.
At the event hosted on August 24, the University of Maryland research team presented the progress on the predictive modeling project over the past year. The project continues to pursue its original goal to allow for local input into the modeling process to address local and regional concerns, such as drug overdoses and crime. The team, led by Ted Knight, is pursuing adding various regional and state level data access to the model, which will increase the overall accuracy of the algorithm by considering diverse sets of activity, law enforcement, behavioral health, and health and environmental data.
“A major goal of this project is to minimize and remove any biases or positive/negative feedback loops–which are generally associated with these models–while maintaining a high degree of accuracy and functionality within the platform,” says Assistant Director of Information Services, John O’Brien. “Developing this model in an open source environment with active input from diverse but collaborative organizations is the best way to achieve this goal. It is exciting to see the regional government, education, law enforcement and health organizations working together to pursue this project as a team.”
The City of Salisbury and the Information Services Department thank Dr. Michael Scott and Lisa Ball of Salisbury University for coordinating the facilities and lunch at Salisbury University, as well as the University of Maryland team and Ted Knight for their collaboration.